Unlocking the Potential of Deep Learning and Parallel Computing in Bioengineering Systems

In the rapidly advancing field of bioengineering, deep learning and parallel computing have emerged as transformative technologies, opening up unprecedented possibilities for research and development. These technologies empower scientists and engineers to tackle complex biological and medical challenges, leading to breakthroughs in disease diagnosis, drug discovery, and personalized medicine.
Deep Learning: A Revolutionary Approach to Biological Data Analysis
Deep learning, a subfield of artificial intelligence, involves training artificial neural networks with multiple hidden layers to extract complex patterns and insights from data. When applied to biological data, deep learning algorithms can identify subtle relationships, detect anomalies, and make predictions with remarkable accuracy.
5 out of 5
Language | : | English |
Hardcover | : | 401 pages |
Item Weight | : | 2.16 pounds |
Dimensions | : | 8.27 x 10.98 inches |
File size | : | 61433 KB |
Text-to-Speech | : | Enabled |
Enhanced typesetting | : | Enabled |
Print length | : | 256 pages |
In bioengineering, deep learning has been successfully employed in tasks such as:
- Disease diagnosis by analyzing medical images (e.g., MRI, CT scans)
- Drug discovery by predicting candidate molecule properties and interactions
- Biomarker discovery by identifying relevant gene expression patterns
- Predictive modeling of disease progression and treatment outcomes
Parallel Computing: Accelerating Biological Simulations and Data Analysis
Parallel computing involves distributing computational tasks across multiple processors or computers, enabling the simultaneous execution of complex operations. This approach significantly reduces processing times, making it essential for large-scale biological simulations and data analysis.
In bioengineering, parallel computing has been widely used for:
- Molecular dynamics simulations to study protein folding and drug binding
- Genome sequencing and assembly for personalized medicine
- High-throughput image analysis for cell counting and classification
- Neuroimaging data analysis for brain mapping and connectivity
Synergistic Integration of Deep Learning and Parallel Computing
The combination of deep learning and parallel computing offers a powerful synergistic effect in bioengineering. Deep learning algorithms can be trained and executed on parallel computing platforms, exploiting the massive computational resources available. This integration enables:
- Training of larger and more complex neural network models
- Faster processing of high-dimensional biological data
- Real-time analysis of streaming data from biosensors and medical devices
- Improved accuracy and reliability in predictive modeling
Applications in Bioengineering Systems
The deep learning and parallel computing environment has revolutionized various bioengineering systems:
- Medical diagnosis and prognosis: AI-assisted imaging diagnostics and predictive modeling enable early detection, accurate diagnosis, and personalized treatment plans.
- Drug discovery and development: Deep learning algorithms accelerate drug molecule design, predict drug efficacy, and optimize drug delivery systems.
- Biomedical device design: Parallel computing facilitates simulations of complex fluid dynamics and tissue mechanics, leading to innovative device designs.
- Personalized medicine: Deep learning and parallel computing power personalized treatment plans based on individual genetic and molecular profiles.
- Biomanufacturing and biotechnology: AI-driven optimization of bioprocesses enhances productivity and efficiency in manufacturing.
Ethical and Societal Implications
While deep learning and parallel computing hold immense potential in bioengineering, it is crucial to consider their ethical and societal implications:
- Data privacy and security: Sensitive biological data requires robust privacy measures and secure storage.
- Bias and fairness: AI algorithms must be trained on representative datasets to avoid biased outcomes.
- Transparency and accountability: The decision-making process of AI models should be transparent and auditable.
- Job displacement: Automation through AI could impact employment in some sectors.
- Access and equity: AI solutions should be accessible to all, regardless of economic or social status.
The integration of deep learning and parallel computing has transformed the landscape of bioengineering, offering unparalleled opportunities for scientific discovery and technological innovation. By harnessing the power of these technologies, scientists and engineers can push the boundaries of knowledge and develop solutions to pressing challenges in healthcare, drug development, and biotechnology. However, it is essential to navigate the ethical and societal implications associated with AI to ensure responsible and equitable use. With careful consideration and continued research, deep learning and parallel computing will continue to revolutionize bioengineering and improve human health and well-being.
5 out of 5
Language | : | English |
Hardcover | : | 401 pages |
Item Weight | : | 2.16 pounds |
Dimensions | : | 8.27 x 10.98 inches |
File size | : | 61433 KB |
Text-to-Speech | : | Enabled |
Enhanced typesetting | : | Enabled |
Print length | : | 256 pages |
Do you want to contribute by writing guest posts on this blog?
Please contact us and send us a resume of previous articles that you have written.
Book
Novel
Page
Chapter
Text
Story
Genre
Reader
Library
Paperback
E-book
Magazine
Newspaper
Paragraph
Sentence
Bookmark
Shelf
Glossary
Bibliography
Foreword
Preface
Synopsis
Annotation
Footnote
Manuscript
Scroll
Codex
Tome
Bestseller
Classics
Library card
Narrative
Biography
Autobiography
Memoir
Reference
Encyclopedia
Franz Rosenzweig
Tatsu Takeuchi
Fotios Tjoumakaris
David Owen
Ward Farnsworth
Russell Friedman
Tina Overton
Vinayak Shinde
Karen Mccall
Klaus Friedrich
Ana Lucia Araujo
Marah Archer
John Block Friedman
Kadiatu Kanneh Mason
Paul Bingley
Lindsay Waller
Stephen J Cimbala
Yves Engler
Josh Peck
The Xerces Society
Light bulbAdvertise smarter! Our strategic ad space ensures maximum exposure. Reserve your spot today!
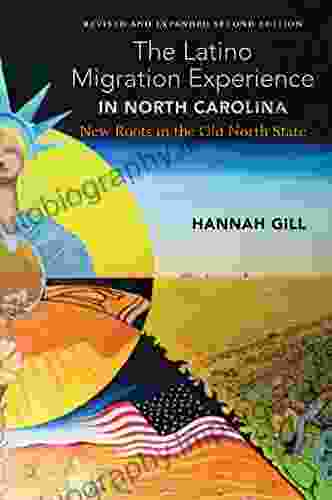

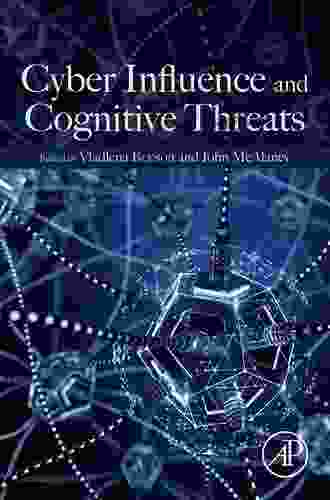

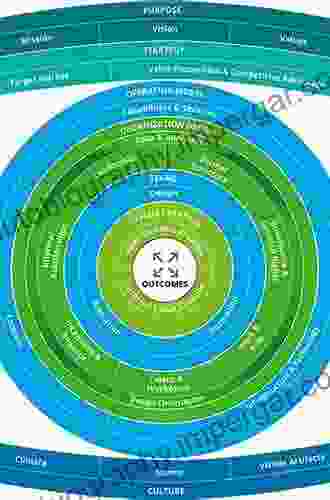

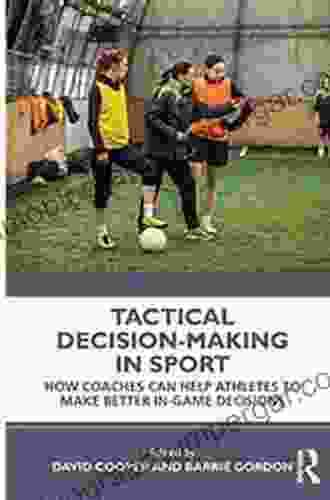

- Spencer PowellFollow ·15.1k
- Benji PowellFollow ·6.4k
- Gene SimmonsFollow ·5.1k
- Eddie PowellFollow ·17k
- Colton CarterFollow ·3.7k
- Dylan MitchellFollow ·5.9k
- Isaiah PowellFollow ·17.2k
- Ethan GrayFollow ·3.8k


Bookkeeping Essentials: How to Succeed as a Bookkeeper
Bookkeeping is the process...
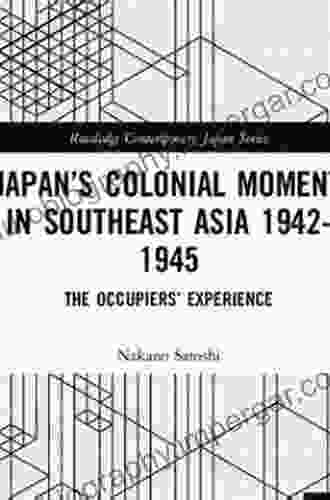

Unveiling the Unseen: The Occupiers Experience - A...
In the vibrant tapestry of contemporary...
5 out of 5
Language | : | English |
Hardcover | : | 401 pages |
Item Weight | : | 2.16 pounds |
Dimensions | : | 8.27 x 10.98 inches |
File size | : | 61433 KB |
Text-to-Speech | : | Enabled |
Enhanced typesetting | : | Enabled |
Print length | : | 256 pages |